Advantages of Multi-Target Drug Discovery
The new platform, called POLYGON, is unique among AI tools for drug discovery in that it can identify molecules with multiple targets, while existing drug discovery protocols currently prioritize single target therapies. Multi-target drugs are of major interest to doctors and scientists because of their potential to deliver the same benefits as combination therapy, in which several different drugs are used together to treat cancer, but with fewer side effects.
“It takes many years and millions of dollars to find and develop a new drug, especially if we’re talking about one with multiple targets.” said Ideker. “The rare few multi-target drugs we do have were discovered largely by chance, but this new technology could help take chance out of the equation and kickstart a new generation of precision medicine.”
How POLYGON Works
The researchers trained POLYGON on a database of over a million known bioactive molecules containing detailed information about their chemical properties and known interactions with protein targets. By learning from patterns found in the database, POLYGON is able to generate original chemical formulas for new candidate drugs that are likely to have certain properties, such as the ability to inhibit specific proteins.
“Just like AI is now very good at generating original drawings and pictures, such as creating pictures of human faces based off desired properties like age or sex, POLYGON is able to generate original molecular compounds based off of desired chemical properties,” said Ideker. “In this case, instead of telling the AI how old we want our face to look, we’re telling it how we want our future drug to interact with disease proteins.”
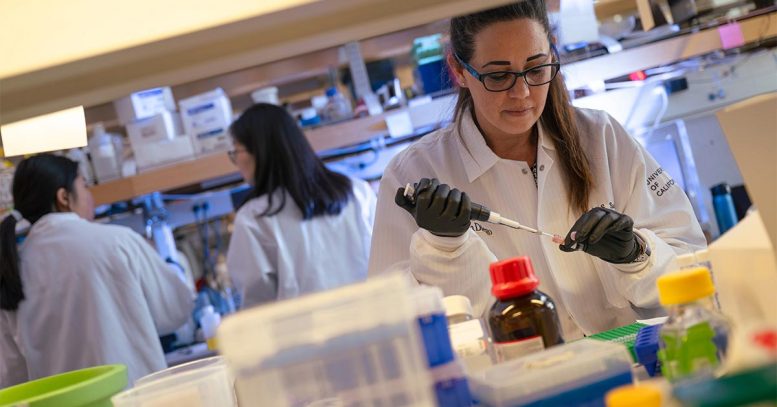
Study co-author Katherine Licon, photographed here at the bench, is lab manager for the Ideker Lab at UC San Diego, which combines computational and traditional wet-lab techniques to answer fundamental questions about disease biology and discover new ways to enhance precision medicine. Credit: Erik Jepsen/UC San Diego
Testing and Results
To put POLYGON to the test, the researchers used it to generate hundreds of candidate drugs that target various pairs of cancer-related proteins. Of these, the researchers synthesized 32 molecules that had the strongest predicted interactions with the MEK1 and mTOR proteins, a pair of cellular signaling proteins that are a promising target for cancer combination therapy. These two proteins are what scientists call synthetically lethal, which means that inhibiting both together is enough to kill cancer cells even if inhibiting one alone is not.
The researchers found that the drugs they synthesized had significant activity against MEK1 and mTOR, but had few off-target reactions with other proteins. This suggests that one or more of the drugs identified by POLYGON could be able to target both proteins as a cancer treatment, providing a list of choices for fine-tuning by human chemists.
“Once you have the candidate drugs, you still need to do all the other chemistry it takes to refine those options into a single, effective treatment,” said Ideker. “We can’t and shouldn’t try to eliminate human expertise from the drug discovery pipeline, but what we can do is shorten a few steps of the process.”
Future of AI in Drug Discovery
Despite this caution, the researchers are optimistic that the possibilities of AI for drug discovery are only just being explored.